
Advantages of feature elimination methods include simplicity and maintaining interpretability of your variables.Īs a disadvantage, though, you gain no information from those variables you’ve dropped.

In the GDP example above, instead of considering every single variable, we might drop all variables except the three we think will best predict what the U.S.’s gross domestic product will look like. Somewhat unsurprisingly, reducing the dimension of the feature space is called “ dimensionality reduction.” There are many ways to achieve dimensionality reduction, but most of these techniques fall into one of two classes:įeature elimination is what it sounds like: we reduce the feature space by eliminating features. are no longer concerns - but we’re moving in the right direction!) (Note: This doesn’t immediately mean that overfitting, etc. You might ask the question, “How do I take all of the variables I’ve collected and focus on only a few of them?” In technical terms, you want to “reduce the dimension of your feature space.” By reducing the dimension of your feature space, you have fewer relationships between variables to consider and you are less likely to overfit your model. Do you understand the relationships between each variable? Do you have so many variables that you are in danger of overfitting your model to your data or that you might be violating assumptions of whichever modeling tactic you’re using? If you’ve worked with a lot of variables before, you know this can present problems. TL DR - you have a lot of variables to consider. Despite being an overwhelming number of variables to consider, this just scratches the surface. You could gather stock price data, the number of IPOs occurring in a year, and how many CEOs seem to be mounting a bid for public office. You know how many members of the House and Senate belong to each political party. Census data from 2010 estimating how many Americans work in each industry and American Community Survey data updating those estimates in between each census. You have any publicly-available economic indicator, like the unemployment rate, inflation rate, and so on. GDP for the entirety of 2016, 2015, and so on. GDP for the first quarter of 2017, the U.S. You have lots of information available: the U.S.
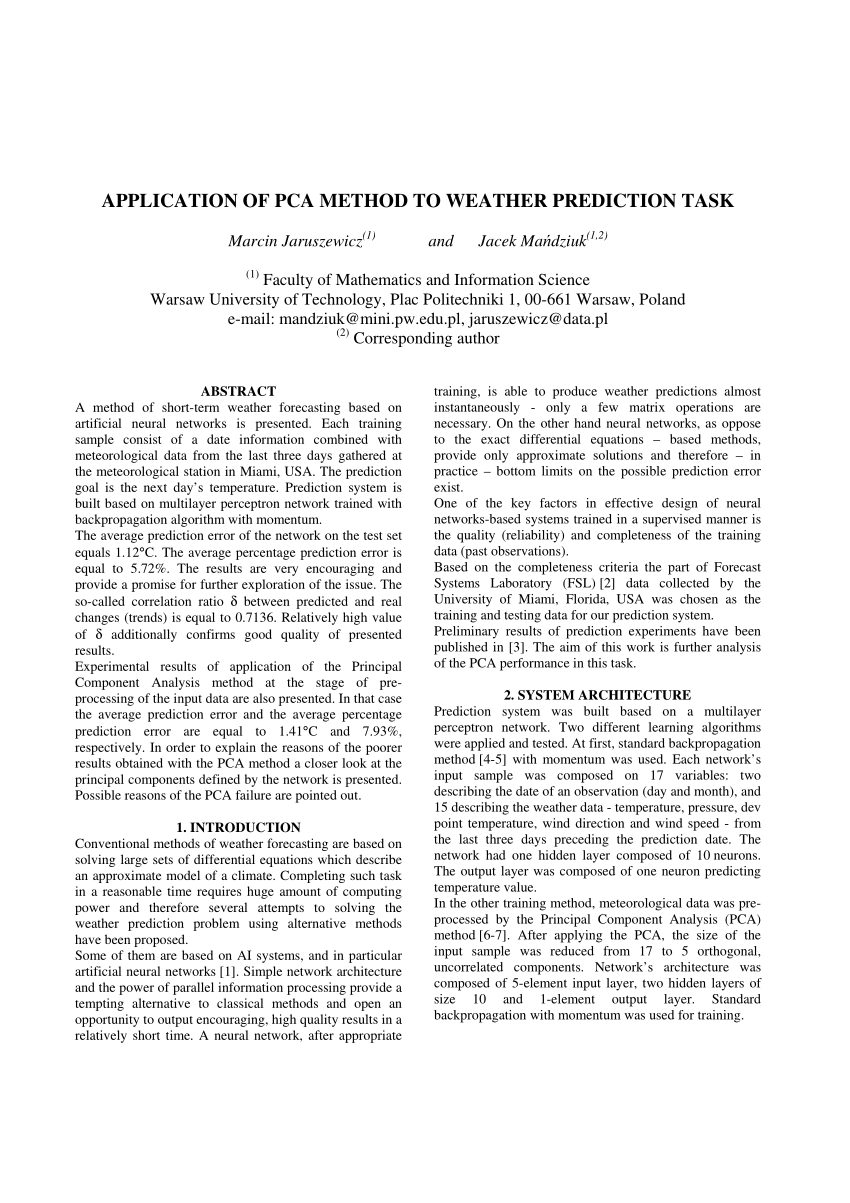
Let’s say that you want to predict what the gross domestic product (GDP) of the United States will be for 2017. I’ve embedded links to illustrations of these topics throughout the article, but hopefully these will serve as a reminder rather than required reading to get through the article. Being familiar with some or all of the following will make this article and PCA as a method easier to understand: matrix operations/linear algebra (matrix multiplication, matrix transposition, matrix inverses, matrix decomposition, eigenvectors/eigenvalues) and statistics/machine learning (standardization, variance, covariance, independence, linear regression, feature selection). While I want to make PCA as accessible as possible, the algorithm we’ll cover is pretty technical. Specifically, I want to present the rationale for this method, the math under the hood, some best practices, and potential drawbacks to the method. It’s safe to say that I’m not “entirely satisfied with the available texts” here.Īs a result, I wanted to put together the “What,” “When,” “How,” and “Why” of PCA as well as links to some of the resources that can help to further explain this topic. Principal component analysis (PCA) is an important technique to understand in the fields of statistics and data science… but when putting a lesson together for my General Assembly students, I found that the resources online were too technical, didn’t fully address our needs, and/or provided conflicting information.
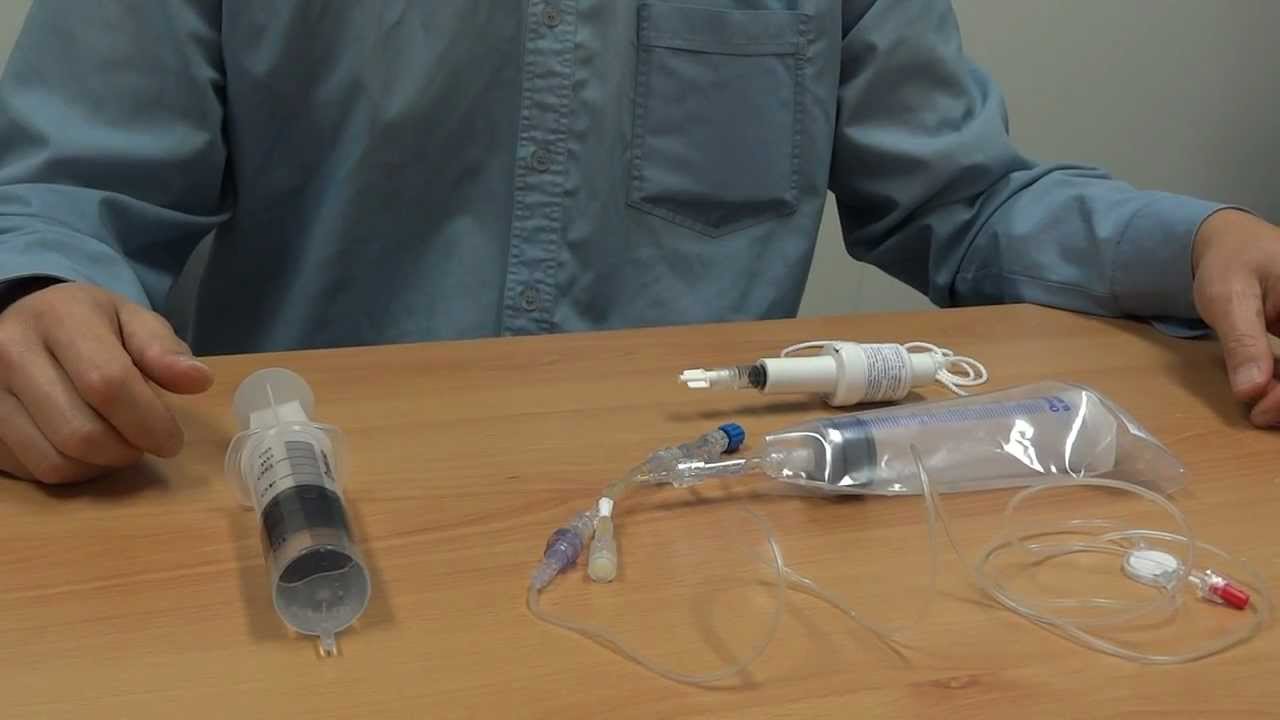
You are writing a book because you are not entirely satisfied with the available texts.” The first is “Why are you writing a book?” and the second is “How is your book different from what’s out there?” The first question is fairly easy to answer.
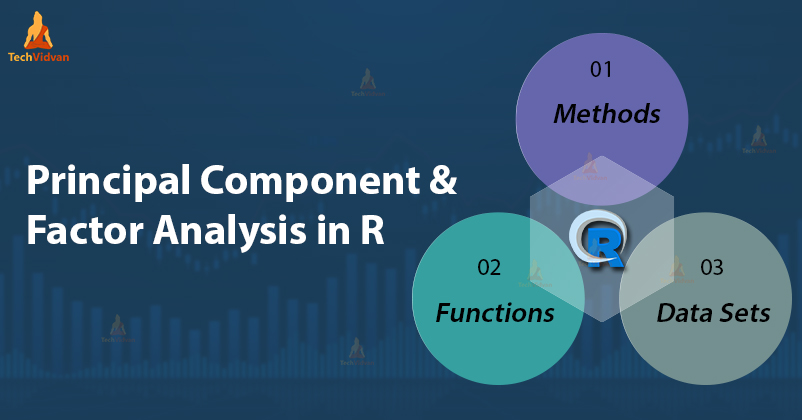
“When someone discovers that you are writing a textbook, one or both of two questions will be asked. A One-Stop Shop for Principal Component AnalysisĪt the beginning of the textbook I used for my graduate stat theory class, the authors (George Casella and Roger Berger) explained in the preface why they chose to write a textbook:
